Dangerous Heavy Metals Found in Vape Pens Even Before Heating
March 21, 2024New Transparent AI Predicts Breast Cancer 5 Years Out New Transparent AI Predicts Breast Cancer 5 Years Out
March 21, 2024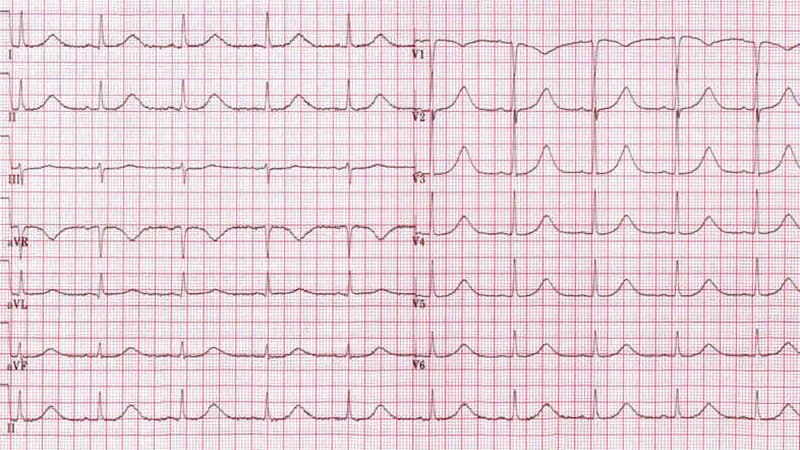
TOPLINE:
A machine learning model was more effective at detecting congenital long QT syndrome on resting ECGs than manual measurement of QT intervals by arrhythmia experts, in a new study.
METHODOLOGY:
- Researchers developed a machine learning approach known as a “convolutional neural network” to detect long QT syndrome on baseline ECGs.
- They tested the model in individuals enrolled in the Canadian National Hearts in Rhythm Organization Registry that includes patients with inherited arrhythmias and cardiomyopathies and their unaffected relatives.
- The study included a broadly representative population-based group of patients with milder forms of long QT syndrome, which can be difficult to identify on a resting ECG. A total of 4521 ECGs from 990 patients were analyzed.
TAKEAWAY:
- Validation within the registry demonstrated the model’s high diagnostic capacity for long QT syndrome detection (AUC, 0.93) and in distinguishing between the two most common genotypes (AUC, 0.91).
- This surpassed expert-measured QTc intervals in detecting long QT syndrome (F1 score, 0.84 vs 0.22) and sensitivity (0.90 vs 0.36), including in patients with normal or borderline QTc intervals.
- Further validation in an external cross-sectional cohort of high-risk patients and genotype-negative controls confirmed these results.
IN PRACTICE:
The researchers explained that long QT syndrome is a cardiac ion channelopathy associated with syncope, ventricular tachycardia, and, rarely, sudden arrhythmic death, but excellent outcomes can be achieved with the correct management. While often detected by QT prolongation on resting ECG, up to half of patients have a normal or borderline QT interval (known as concealed long QT syndrome) on resting ECG. In this study, the convolutional neural network machine learning model was able to detect long QT syndrome with higher F1 scores (a measure of machine learning model’s accuracy) and sensitivities compared with QTc intervals measured by arrhythmia experts. Broader validation over an unselected general population may support broad application of this model to stratify torsade de pointes risk in patients with suspected long QT syndrome. The researchers have made details of their model publicly available to further advance the field.
SOURCE:
The study, led by River Jiang, MD, University of British Columbia, Vancouver, British Columbia, Canada, was published online in JAMA Cardiology on March 6.
LIMITATIONS:
Training of the model used a case-control cohort that included relatives who were referred due to family history of an inherited arrhythmia and were determined to be unaffected. This control cohort was at a low risk for long QT syndrome and easier to distinguish from patients with congenital long QT syndrome.
The second validation cohort involved a higher-risk cohort of patients with even the genome negative control group having had a sufficiently elevated pretest suspicion for long QT syndrome to warrant genetic testing. However, the model still effectively identified long QT syndrome in this cohort (albeit with reduced discriminatory ability) including in patients with normal or only borderline-abnormal QTc intervals on their resting ECGs.
DISCLOSURES:
The National Hearts in Rhythm Organization was funded by the Canadian Institutes of Health Research.